A brief look into using a hybrid GPU/VRAM + CPU/RAM approach to LLM inference with the KTransformers inference library.
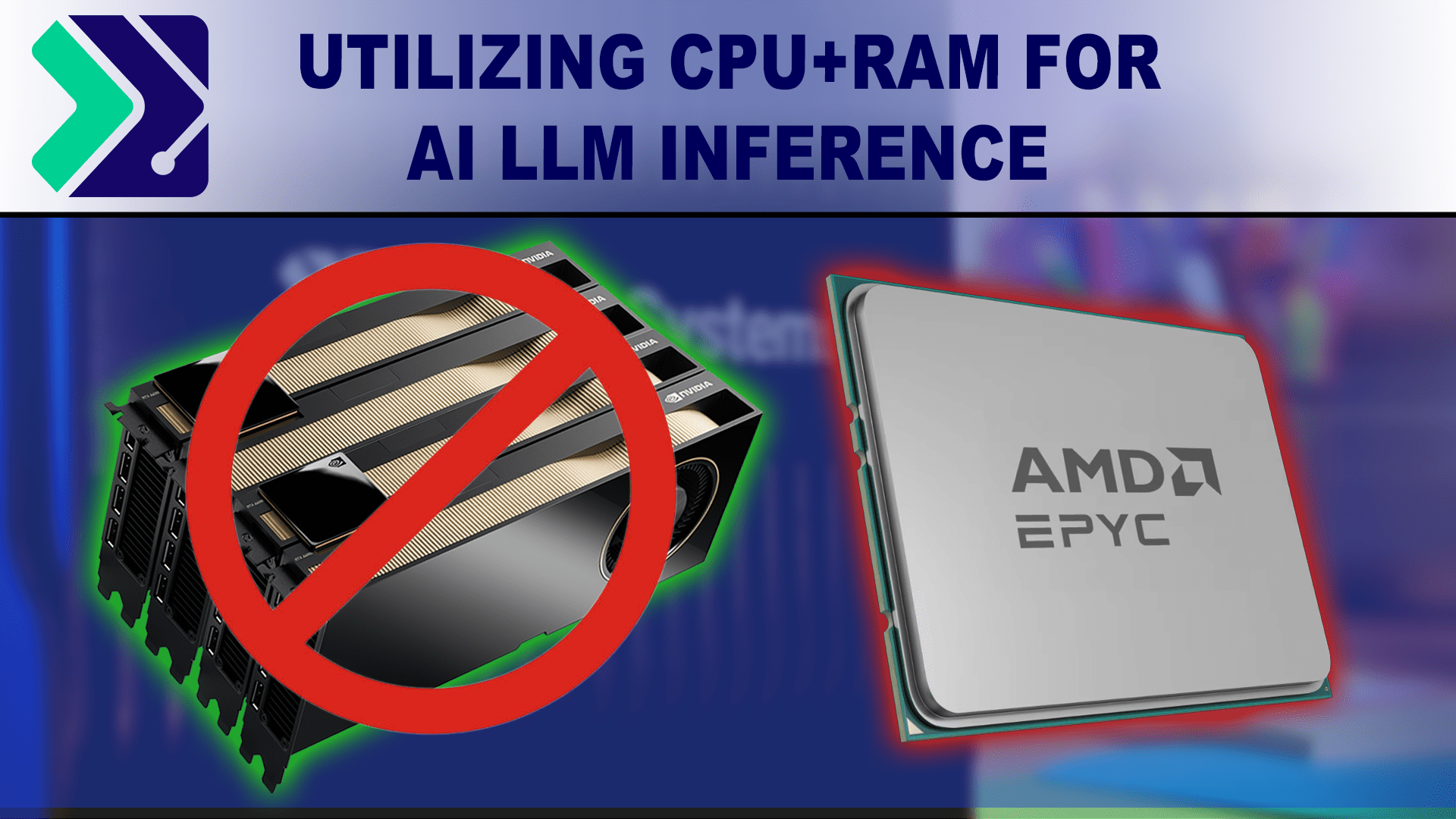
A brief look into using a hybrid GPU/VRAM + CPU/RAM approach to LLM inference with the KTransformers inference library.
An introduction to NPU hardware and its growing presence outside of mobile computing devices.
Presenting local AI-powered software options for tasks such as image & text generation, automatic speech recognition, and frame interpolation.
The performance improvement with the new Zen4 TrPRO over the Zen3 TrPRO is very impressive!
My first recommendation for a Scientific and Engineering workstation CPU would now be the AMD Zen4 architecture as either Zen4 Threadripper PRO or Zen4 EPYC for multi-socket systems.
Evaluating the speed of GeForce RTX 40-Series GPUs using NVIDIA’s TensorRT-LLM tool for benchmarking GPU inference performance.
Results and thoughts with regard to testing a variety of Stable Diffusion training methods using multiple GPUs.
This post is Part 2 in a series on how to configure a system for LLM deployments and development usage. Part 2 is about installing and configuring container tools, Docker and NVIDIA Enroot.
This post is Part 1 in a series on how to configure a system for LLM deployments and development usage. The configuration will be suitable for multi-user deployments and also useful for smaller development systems. Part 1 is about the base Linux server setup.
In this post address the question that’s been on everyone’s mind; Can you run a state-of-the-art Large Language Model on-prem? With *your* data and *your* hardware? At a reasonable cost?
This is a short note on setting up the Apache web server to allow system users to create personal websites and web apps in their home directories.